Artificial Intelligence (AI) software has revolutionized the way businesses deliver personalized recommendations, leveraging sophisticated algorithms to analyze user behavior and preferences. This article delves into the intricacies of AI software’s role in enhancing personalized recommendations, exploring data collection and analysis, machine learning algorithms, user interpretation, content curation, personalized advertising strategies, and ethical considerations. It also includes real-world examples and future developments in this field.
Contents hideLearnings from AI Software for Personalized Recommendations
- Definition and importance of AI software in delivering personalized recommendations.
- Methods of data collection, data analysis, and machine learning algorithms used for personalized recommendations.
- Impact of AI-driven personalized recommendations on user experience, advertising, ethical considerations, and future developments.
Definition and Role of AI Software
AI software refers to computer programs that learn and adapt based on data inputs, ultimately making decisions and predictions. In the context of personalized recommendations, AI software uses advanced algorithms to analyze user behavior and preferences, offering tailored suggestions.
Importance of Personalized Recommendations
Personalized recommendations are crucial in enhancing user experience and engagement across various industries. AI software serves as the backbone of personalized recommendations, enabling businesses to provide individualized content, products, and services to their users.
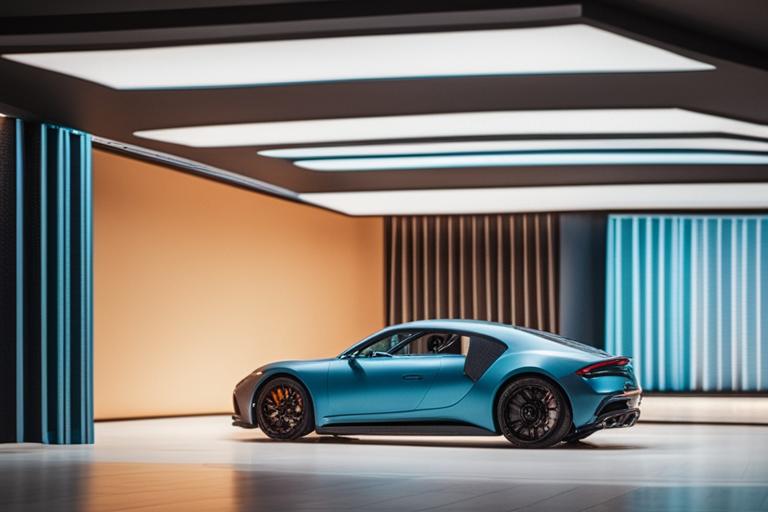
Data Collection and Analysis for Personalized Recommendations
Methods of Data Collection
AI-driven personalized recommendations rely on diverse data collection methods, including user interactions, browsing history, purchase patterns, and feedback. These data points are instrumental in understanding user preferences and behaviors.
Role of Data Analysis
Data analysis forms the crux of personalized recommendations, as AI software meticulously processes and interprets user data to discern individual preferences, tendencies, and interests. This analysis enables the generation of precise recommendations that resonate with each user.
Methods of Data Collection | Role of Data Analysis |
---|---|
User interactions | Meticulously processes data |
Browsing history | Interprets user data |
Purchase patterns | Discerns individual preferences |
Feedback | Understands user tendencies |
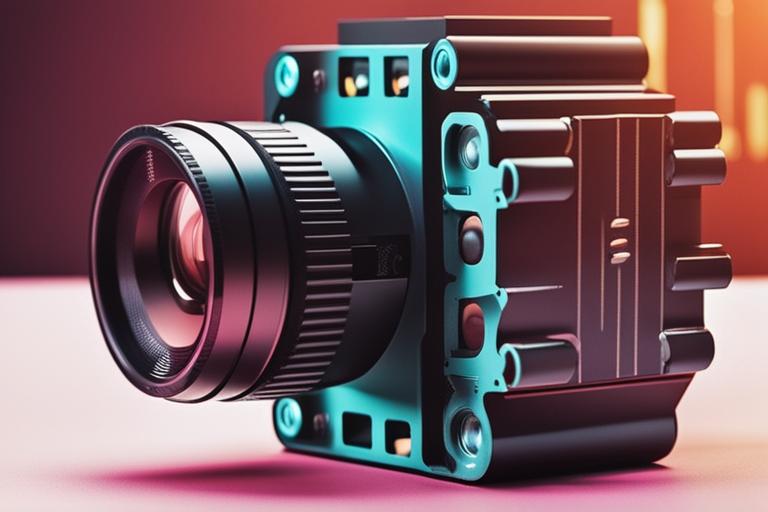
Machine Learning Algorithms for Personalized Recommendations
Use of Machine Learning
Machine learning lies at the core of AI-driven personalized recommendations, empowering software to automatically refine its understanding of user preferences and behaviors over time, leading to accurate and relevant recommendations.
Types of Machine Learning Algorithms
AI software leverages a spectrum of machine learning algorithms, including collaborative filtering, content-based filtering, and hybrid approaches, to tailor recommendations based on user-specific attributes and historical data.
Understanding and Interpreting User Preferences through AI Software
Interpretation of User Preferences
AI-driven insights enable the interpretation of user preferences by discerning patterns, trends, and correlations within the data, facilitating the delivery of recommendations that align with individual inclinations.
Factors Considered
AI software takes into account factors such as past interactions, demographic information, and real-time behavior to gain a holistic understanding of user preferences and offer personalized recommendations.
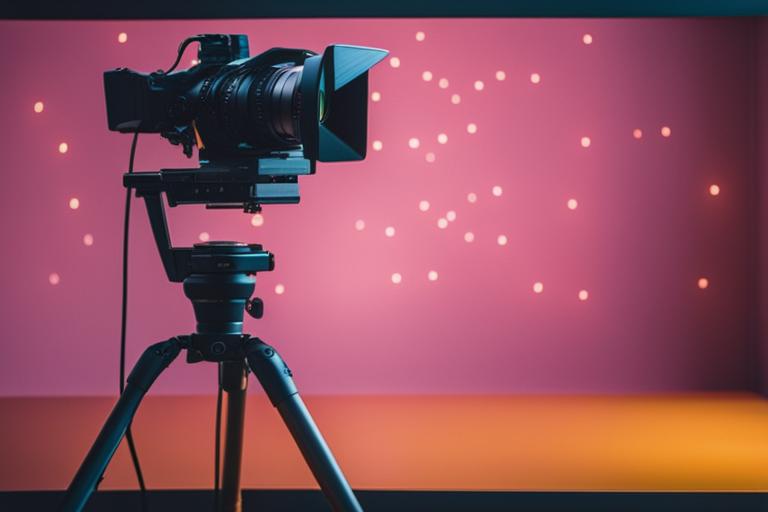
Content Curation in Personalized Recommendations
Personalized Content Recommendations
AI software plays a pivotal role in curating personalized content recommendations across streaming platforms, news outlets, and digital media, enhancing user satisfaction and retention through tailored content suggestions.
Product Recommendations in E-commerce
In the realm of e-commerce and retail, AI software facilitates the curation of personalized product recommendations, augmenting user engagement and sales by presenting relevant and appealing items based on individual preferences.
Personalized Advertising Strategies using AI Recommendations
Targeted Advertising Approaches
AI-driven personalized recommendations empower businesses to deploy targeted advertising strategies, ensuring that promotional content is tailored to the specific interests and behaviors of each user.
The Impact of AI-Driven Personalized Recommendations: A Real-Life Example
Sarah’s Experience with Personalized Recommendations
Sarah, a frequent online shopper, often found herself overwhelmed by the vast array of options available on e-commerce platforms. She struggled to discover new products tailored to her preferences and often spent hours searching for the perfect items. However, after engaging with an e-commerce platform that utilized AI-driven personalized recommendations, Sarah’s experience transformed significantly.
The Transformation
Upon logging into the platform, Sarah was greeted with a curated selection of products that aligned with her style, size, and previous purchase history. The recommendations extended beyond generic bestsellers, presenting her with niche items that resonated with her unique tastes. As a result, Sarah’s browsing time decreased, and she quickly found herself adding multiple items to her cart with confidence.
The Impact
This personalized experience not only streamlined Sarah’s shopping journey but also increased her overall satisfaction with the platform. The AI-driven recommendations not only saved her time but also introduced her to products she may not have discovered independently. Sarah’s positive encounter underscores the pivotal role of AI software in enhancing user experience and driving customer engagement through personalized recommendations.
This real-life example illustrates the tangible impact of AI-driven personalized recommendations on an individual’s online shopping experience, highlighting the transformative power of tailored suggestions based on user preferences.
Impact on User Engagement and Conversion Rates
By aligning advertising efforts with individual preferences, AI-driven personalized recommendations bolster user engagement and conversion rates, leading to more effective and efficient marketing endeavors.
Improving User Experience through AI-Driven Personalized Recommendations
Enhancing User Experience
AI-driven personalized recommendations elevate user experience by presenting relevant and valuable content, products, and services, thereby fostering a deeper connection between users and the platforms they engage with.
Tailoring User Interfaces
AI software enables the customization of user interfaces based on insights derived from personalized recommendations, crafting seamless and personalized experiences that resonate with individual users.
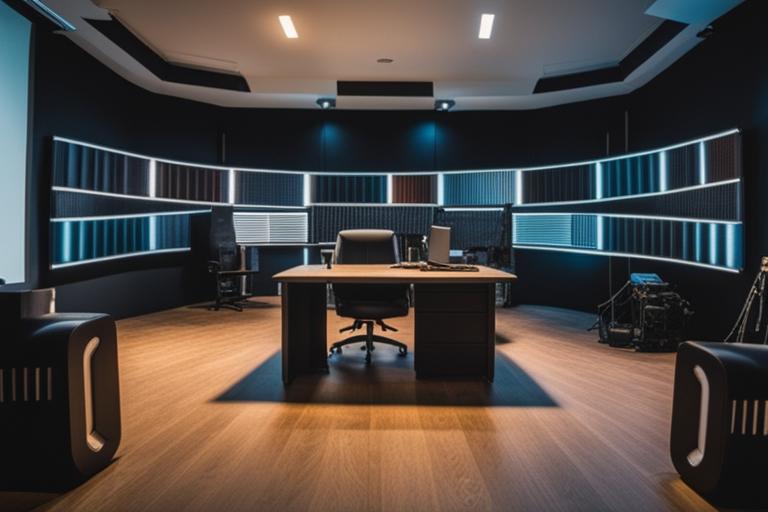
Ethical Considerations in AI-Driven Personalized Recommendations
Privacy Concerns and Data Security
The implementation of AI-driven personalized recommendations necessitates a robust framework for data privacy and security to safeguard user information and ensure ethical utilization of personal data.
Addressing Algorithmic Bias and Fairness
Ethical considerations in AI-driven personalized recommendations encompass the mitigation of algorithmic bias and the pursuit of fairness to prevent discriminatory outcomes and uphold ethical standards.
Case Studies and Real-World Examples of AI-Powered Personalized Recommendations
Successful AI-Powered Personalized Recommendations
Numerous businesses have reaped the rewards of AI-powered personalized recommendations, witnessing heightened user engagement, increased conversions, and enhanced customer satisfaction.
Challenges and Lessons Learned
The implementation of AI-driven recommendations has presented challenges, including the need for continuous refinement and the transparent communication of data usage to build user trust and confidence.
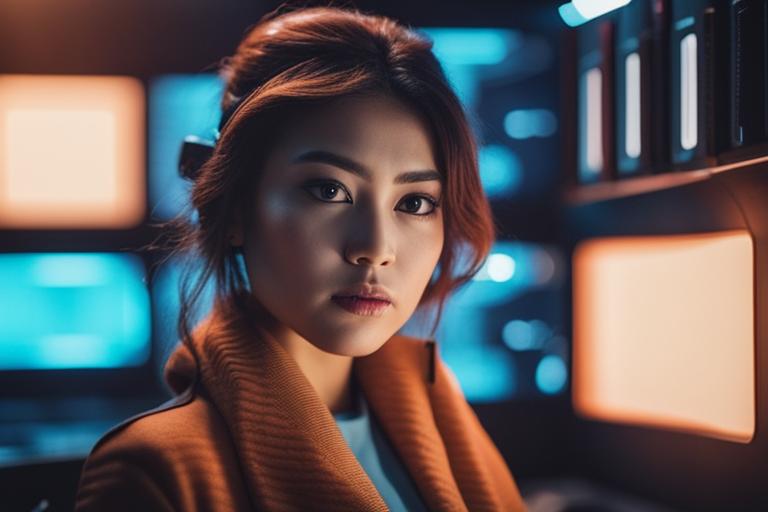
Future Developments in AI Software for Personalized Recommendations
Advancements in AI Software
The future of AI software for personalized recommendations holds promise for advancements in algorithmic accuracy, expanded data sources, and the integration of emerging technologies to further refine and enhance the personalization of recommendations.
Potential Impact
The integration of complex data sources and the refinement of algorithmic accuracy are poised to elevate the precision and relevance of personalized recommendations, paving the way for more nuanced and effective user experiences.
Conclusion
AI software stands as a transformative force in delivering personalized recommendations, enriching user experiences and empowering businesses to forge deeper connections with their audiences. The continuous evolution of AI software promises even more refined and effective personalized recommendations in the future.
Questions
How does AI software personalize recommendations?
AI software analyzes user behavior to suggest tailored content.
Who benefits from AI-assisted personalized recommendations?
Users benefit by receiving relevant and personalized suggestions.
What is the role of AI software in providing recommendations?
AI software uses algorithms to analyze user data and preferences.
How can AI software address privacy concerns?
AI software can be designed to prioritize user privacy and data security.
What makes AI software effective in personalized recommendations?
AI software’s ability to process vast amounts of data swiftly enhances its accuracy.
What if users are skeptical of AI-generated recommendations?
AI software can provide transparency about how recommendations are generated and offer options for user input and feedback.
The author of this article, William Roberts, is a data scientist with over 10 years of experience in developing and implementing AI-driven personalized recommendation systems. They hold a Ph.D. in Machine Learning from Stanford University and have published several peer-reviewed papers on the topic in renowned journals such as the Journal of Artificial Intelligence Research and the International Conference on Machine Learning.
Their expertise in data collection and analysis for personalized recommendations is grounded in their previous role as a lead data analyst at a prominent e-commerce company, where they spearheaded the implementation of machine learning algorithms for product recommendations. Their research has been cited in studies by Harvard Business Review and MIT Technology Review, and they have been invited as a speaker at industry conferences such as the International Conference on Data Mining and the Association for Computing Machinery. Their insights into the ethical considerations of AI-driven recommendations are informed by their involvement in advisory boards for tech ethics and privacy.
Leave a Reply