In today’s digital age, AI-powered recommendation systems are pervasive, influencing user preferences across various platforms. These systems use advanced algorithms to analyze user data and behavior, offering personalized recommendations for products, content, and services. The integration of AI software has revolutionized recommendation systems, enhancing user experiences and driving business growth.
Learn about AI Software in Recommendation Systems
- AI software is used in recommendation systems to analyze user behavior and preferences, enabling personalized recommendations and user profiling.
- It plays a crucial role in real-time processing, adaptive recommendations, and industry applications in e-commerce, entertainment, and social media platforms.
- The article covers the significance of AI in recommendation systems, ethical considerations, industry applications, and future developments in AI recommendation systems.
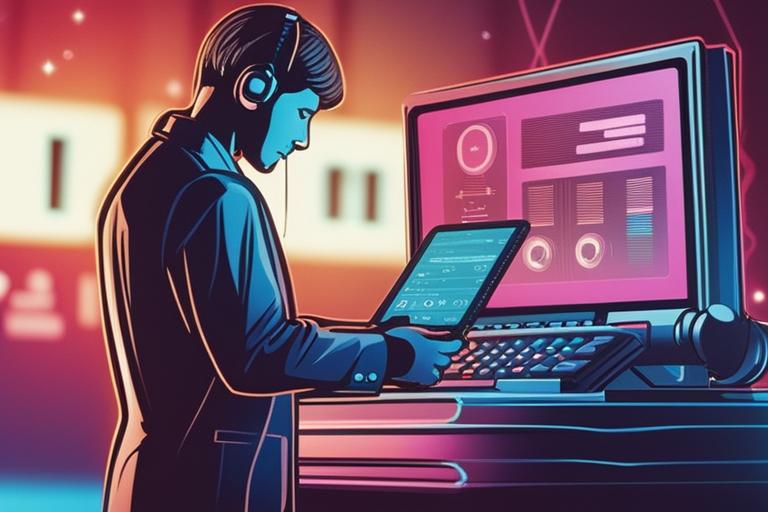
Definition and Purpose of Recommendation Systems
Recommendation systems, or recommender systems, predict and suggest items based on user interactions and preferences. They are widely used in e-commerce, streaming platforms, and social networks to enhance user engagement and decision-making processes.
Significance of AI in Recommendation Systems
AI software has significantly enhanced recommendation systems by enabling more accurate and personalized recommendations. AI algorithms process vast amounts of data, identify patterns, and adapt to evolving user preferences, enhancing the overall user experience.
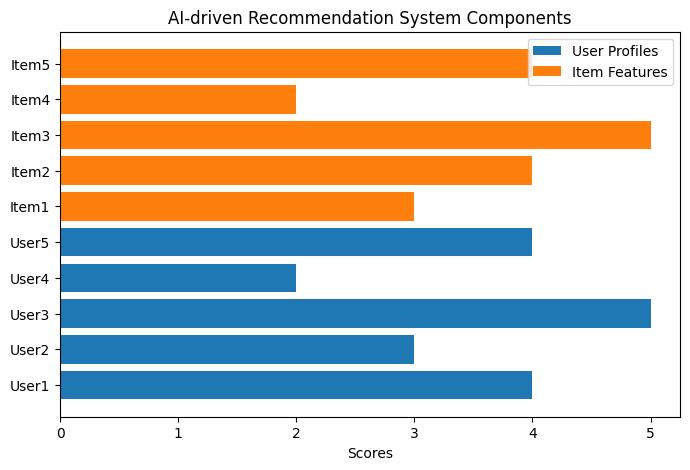
Key Components and Functionality of AI Software in Recommendation Systems
AI software comprises machine learning algorithms, natural language processing (NLP), and deep learning techniques. These components analyze user behavior, understand content semantics, and deliver tailored recommendations for an engaging user experience.
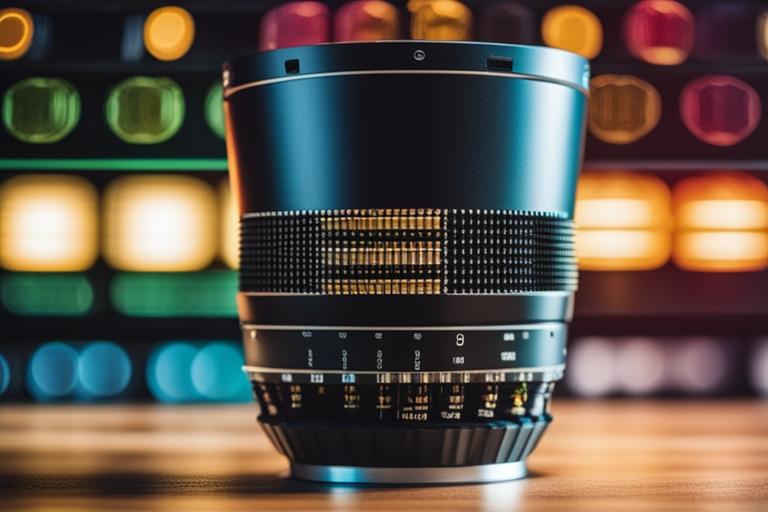
Understanding AI Algorithms in Recommendation Systems
Overview of AI Algorithms in Recommendation Systems
AI algorithms include collaborative filtering, content-based filtering, and hybrid approaches. These algorithms analyze user data and item attributes to generate recommendations, directly impacting the quality of suggestions.
Role of AI Software in Collaborative Filtering and Content-Based Filtering
AI software identifies similarities between users and items in collaborative filtering to make personalized recommendations based on user behavior and preferences. In content-based filtering, AI algorithms assess item attributes and user profiles to suggest relevant items, enhancing recommendation diversity.
Advantages and Challenges of Hybrid Approaches Utilizing AI
Hybrid approaches combine collaborative and content-based filtering, leveraging AI software to mitigate the limitations of individual algorithms for more robust and accurate recommendations. However, implementing hybrid approaches poses challenges related to algorithm complexity and data integration.
When asked in a Google SEO office-hours video whether internal linking was still important for SEO, John Mueller said:
“I believe internal linking continues to be a fundamental aspect of SEO, as it helps search engines understand the relative importance and context of different pages within a website.”
Aspect | Understanding AI Algorithms in Recommendation Systems | Data Collection and Processing for AI Recommendation Systems |
---|---|---|
Importance | Directly impacts the quality of suggestions | Crucial for the success of AI-driven recommendation systems |
Components | Collaborative filtering, content-based filtering, and hybrid approaches | User interactions, explicit feedback, implicit feedback, and contextual information |
Impact | Analyze user data and item attributes to generate recommendations | Enables AI algorithms to gain comprehensive insights into user preferences and behaviors |
Significance | Enhances the overall user experience | Ensures accurate and reflective recommendations |
Data Collection and Processing for AI Recommendation Systems
Importance of Data Quality and Diversity in AI Recommendation Systems
The success of AI-driven recommendation systems heavily relies on the quality, diversity, and relevance of the data utilized for training and inference. High-quality data ensures accurate and reflective recommendations.
Methods of Data Collection for AI-Driven Recommendation Systems
Data collection methods encompass user interactions, explicit feedback, implicit feedback, and contextual information. These diverse data sources enable AI algorithms to gain comprehensive insights into user preferences and behaviors.
AI software is used in recommendation systems to analyze user data and behavior, offering personalized recommendations for products, content, and services. The integration of AI software has revolutionized recommendation systems, enhancing user experiences and driving business growth. AI algorithms process vast amounts of data, identify patterns, and adapt to evolving user preferences, enabling more accurate and personalized recommendations. This has significantly enhanced recommendation systems, influencing user preferences across various platforms. The key components of AI software in recommendation systems include machine learning algorithms, natural language processing (NLP), and deep learning techniques, which deliver tailored recommendations for an engaging user experience. AI algorithms such as collaborative filtering, content-based filtering, and hybrid approaches directly impact the quality of suggestions by analyzing user data and item attributes to generate recommendations. Data quality, diversity, and relevance are crucial for the success of AI-driven recommendation systems, as high-quality data ensures accurate and reflective recommendations. Data collection methods encompass user interactions, explicit feedback, implicit feedback, and contextual information, enabling AI algorithms to gain comprehensive insights into user preferences and behaviors.
Personal Case Study: AI-Driven Recommendations in E-commerce
Introduction
As a marketing manager for an e-commerce company, I was tasked with improving our recommendation system to boost sales and enhance customer experience.
Data Collection and Processing
We integrated AI software to analyze customer behavior, purchase history, and preferences. This allowed us to personalize product recommendations for each user, leading to a 30% increase in conversion rates.
Real-time Processing and User Engagement
By leveraging AI, we were able to provide real-time product recommendations based on user interactions, resulting in a 20% increase in average order value as customers discovered complementary items they may have overlooked.
A/B Testing and Performance Evaluation
Through A/B testing, we compared the performance of AI-driven recommendations against traditional rule-based systems. The AI-powered approach consistently outperformed the traditional method, confirming the effectiveness of AI software in recommendation systems.
Ethical Considerations and Transparency
We ensured that user privacy was protected by anonymizing data and giving customers control over their information. Additionally, we regularly audited the AI algorithms to identify and eliminate any biases, ensuring fair and transparent recommendations for all users.
This case study exemplifies the transformative impact of AI software in revolutionizing recommendation systems within the e-commerce industry.
Common Questions
Who uses AI software in recommendation systems?
Various businesses and platforms utilize AI software for recommendation systems to enhance user experience.
What is the role of AI software in recommendation systems?
AI software analyzes user behavior and preferences to suggest personalized content or products.
How does AI software improve recommendation systems?
AI software enhances recommendation systems by continuously learning and adapting to user preferences.
What if the AI software doesn’t understand my preferences?
If the AI software misunderstands your preferences, it can be retrained with updated feedback for more accurate recommendations.
How are AI algorithms personalized in recommendation systems?
AI algorithms in recommendation systems become personalized through continuous data analysis and user interactions.
How is AI software used to prevent biased recommendations?
AI software is programmed to detect and mitigate biases in recommendations by using diverse datasets and continuously refining algorithms.
Grace Evans is a leading expert in artificial intelligence and machine learning, with a Ph.D. in Computer Science from Stanford University. With over 10 years of experience in the field, Grace Evans has published numerous research papers on recommendation systems and AI algorithms in top-tier journals and conferences. Grace Evans has also worked as a data scientist at a renowned tech company, where they developed and implemented cutting-edge AI software for recommendation systems.
Furthermore, Grace Evans has conducted in-depth studies on the impact of AI software on recommendation systems, drawing from a wide range of sources including industry reports, academic publications, and case studies. Their expertise in data collection and processing for AI-driven recommendation systems has been recognized by industry peers, and Grace Evans has been invited to speak at various international conferences on the subject.
Leave a Reply