Artificial Intelligence (AI) has significantly impacted various industries, but concerns about potential biases in AI systems and their influence on decision-making processes have escalated. This article explores crucial measures for preventing AI bias, including the sources of bias, prevention strategies, ethical considerations, regulatory frameworks, industry best practices, case studies, future outlook, user education, and collaboration initiatives.
Learn about AI Bias Prevention
By reading this article, you will learn:
– Methods for ensuring fairness in AI
– Data collection and preprocessing
– Algorithm design and evaluation
– The ethical considerations and industry best practices for AI bias mitigation
What Measures Are Taken to Ensure AI Software Is Not Biased?
Artificial Intelligence (AI) has significantly impacted various industries, but concerns about potential biases in AI systems and their influence on decision-making processes have escalated. This article explores crucial measures for preventing AI bias, including the sources of bias, prevention strategies, ethical considerations, regulatory frameworks, industry best practices, case studies, future outlook, user education, and collaboration initiatives.
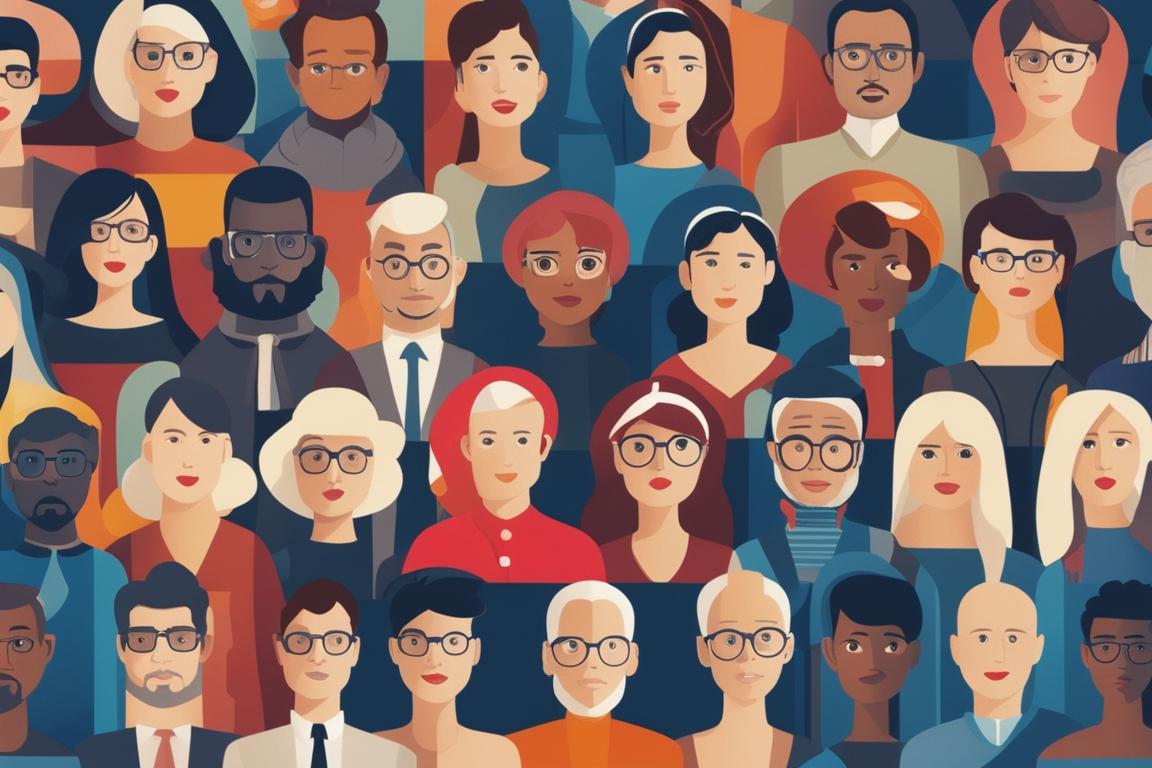
Understanding Bias in AI
Definition of Bias in AI
Bias in AI refers to systematic and unfair preferences or prejudices unintentionally integrated into AI systems during development, training, or implementation. These biases can lead to discriminatory outcomes, undermining fairness and accuracy.
Impact of Bias in AI Decision Making
Biases in AI can perpetuate societal inequalities, reinforce stereotypes, and adversely affect underrepresented groups. This can result in discriminatory practices in areas such as hiring, lending, and law enforcement, eroding trust in AI systems.
Importance of Mitigating AI Bias
Mitigating AI bias is crucial for fostering trust in AI technologies, ensuring equitable treatment, and upholding ethical standards in algorithmic decision-making.
Strategies for Minimizing Bias in Training Data | Description |
---|---|
Data Anonymization | Anonymizing sensitive attributes in training data, such as race, gender, or age, can reduce the risk of propagating biases in AI systems. |
Diverse Data Sampling | Adopting diverse and representative data sampling techniques ensures that AI models are trained on a balanced and inclusive set of examples, reducing the likelihood of biased outcomes. |
Identifying and Mitigating Potential Sources of Bias | Thoroughly examining training data for potential sources of bias and implementing corrective measures is essential for minimizing the impact of bias on AI decision-making processes. |
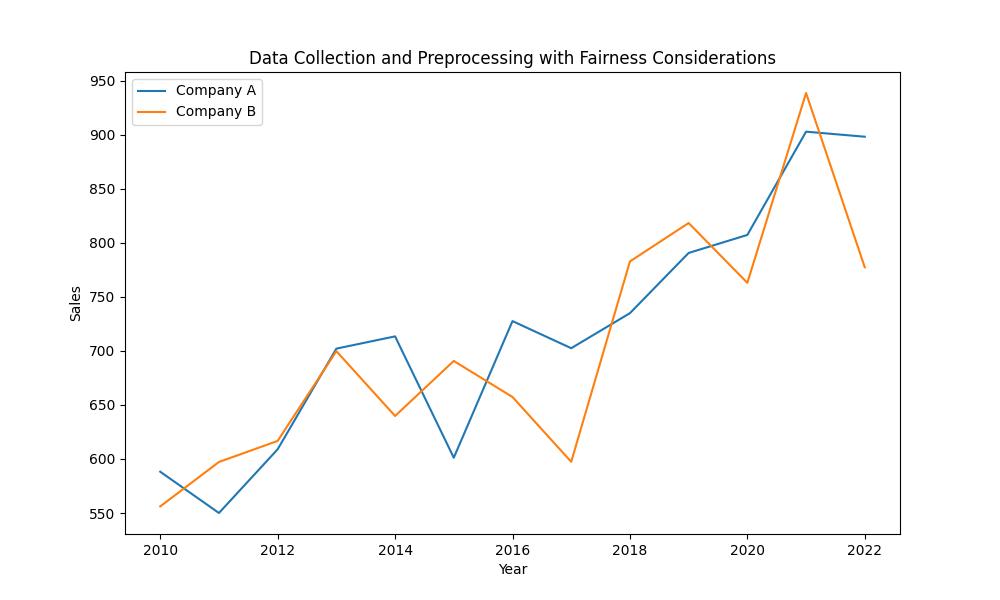
Data Collection and Preprocessing for Fairness in AI
Importance of Careful Data Selection
Careful selection of training data sets is crucial to mitigate bias, as biased data can perpetuate prejudices and stereotypes, leading to skewed outcomes.
Strategies for Minimizing Bias in Training Data
- Data Anonymization: Anonymizing sensitive attributes in training data, such as race, gender, or age, can reduce the risk of propagating biases in AI systems.
- Diverse Data Sampling: Adopting diverse and representative data sampling techniques ensures that AI models are trained on a balanced and inclusive set of examples, reducing the likelihood of biased outcomes.
- Identifying and Mitigating Potential Sources of Bias: Thoroughly examining training data for potential sources of bias and implementing corrective measures is essential for minimizing the impact of bias on AI decision-making processes.
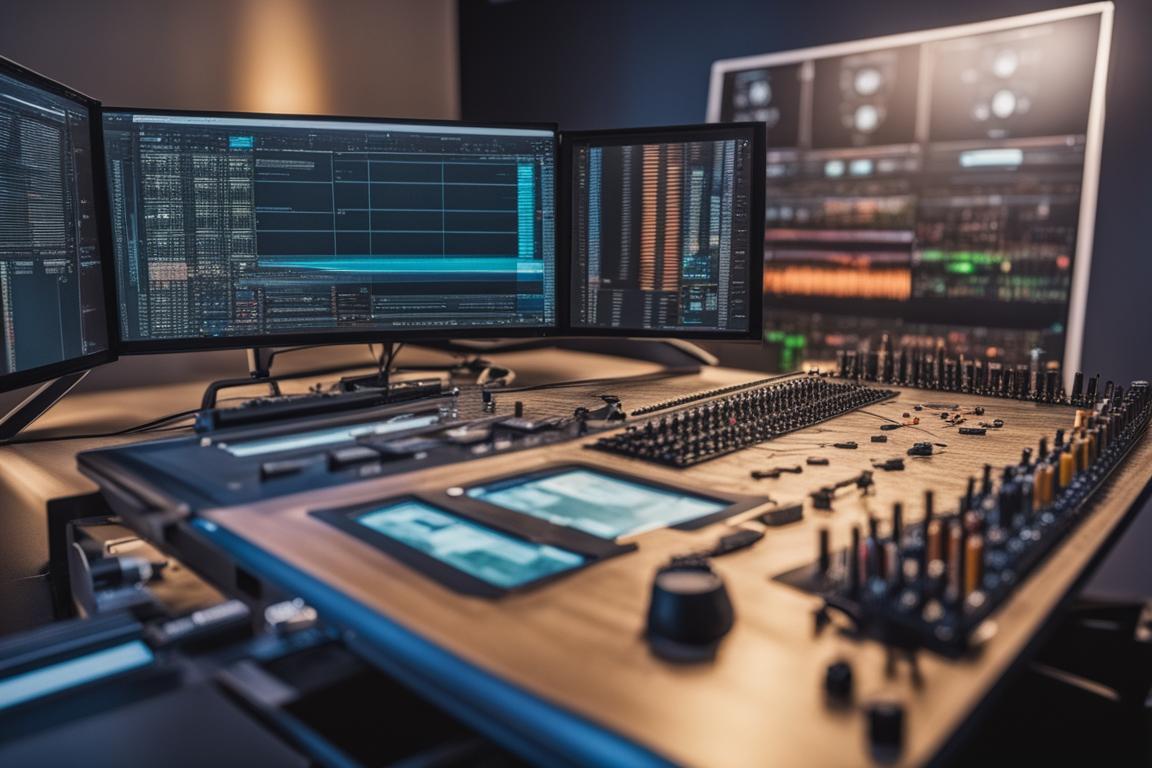
Algorithm Design and Evaluation for Fairness
Fairness Constraints in AI Algorithms
Integrating fairness constraints into AI algorithm design prevents discriminatory outcomes and promotes equitable treatment across different demographic groups.
Bias Detection Metrics
Using bias detection metrics helps assess the fairness of AI algorithms, identify potential bias, and guide model refinement to ensure equitable performance.
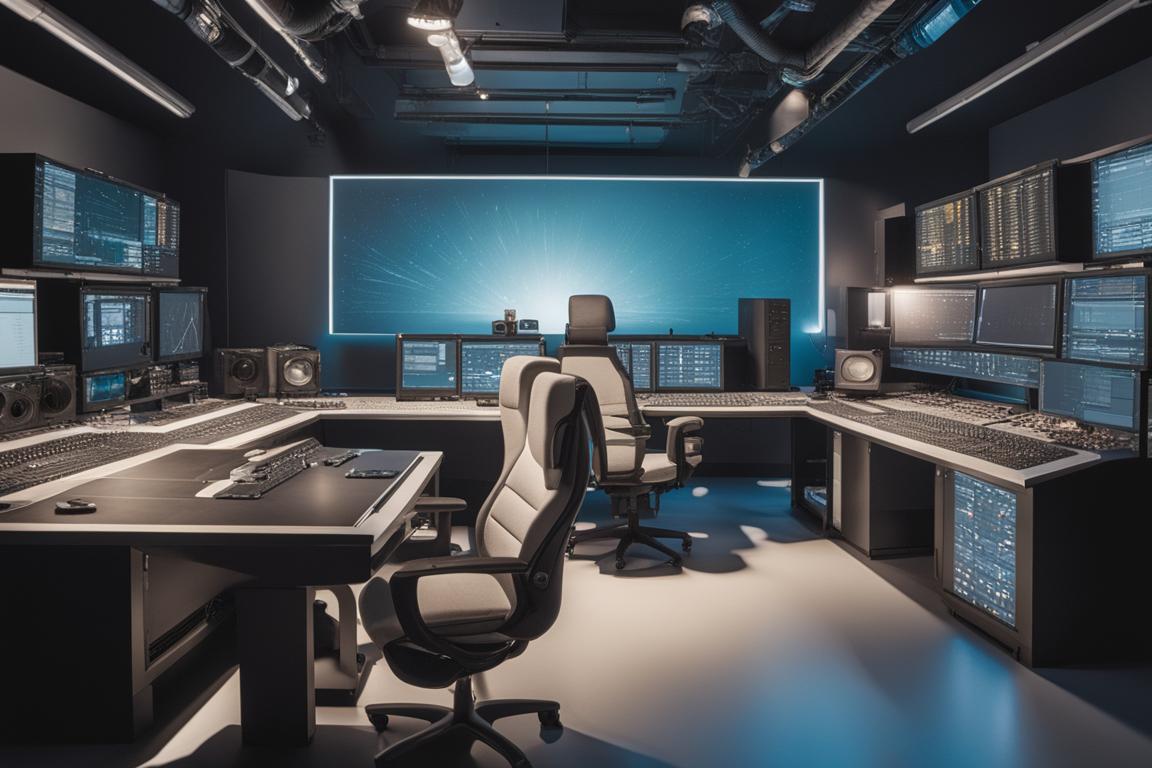
Techniques for Model Interpretability
Incorporating techniques for model interpretability enables stakeholders to understand AI system decision-making processes and rectify biases.
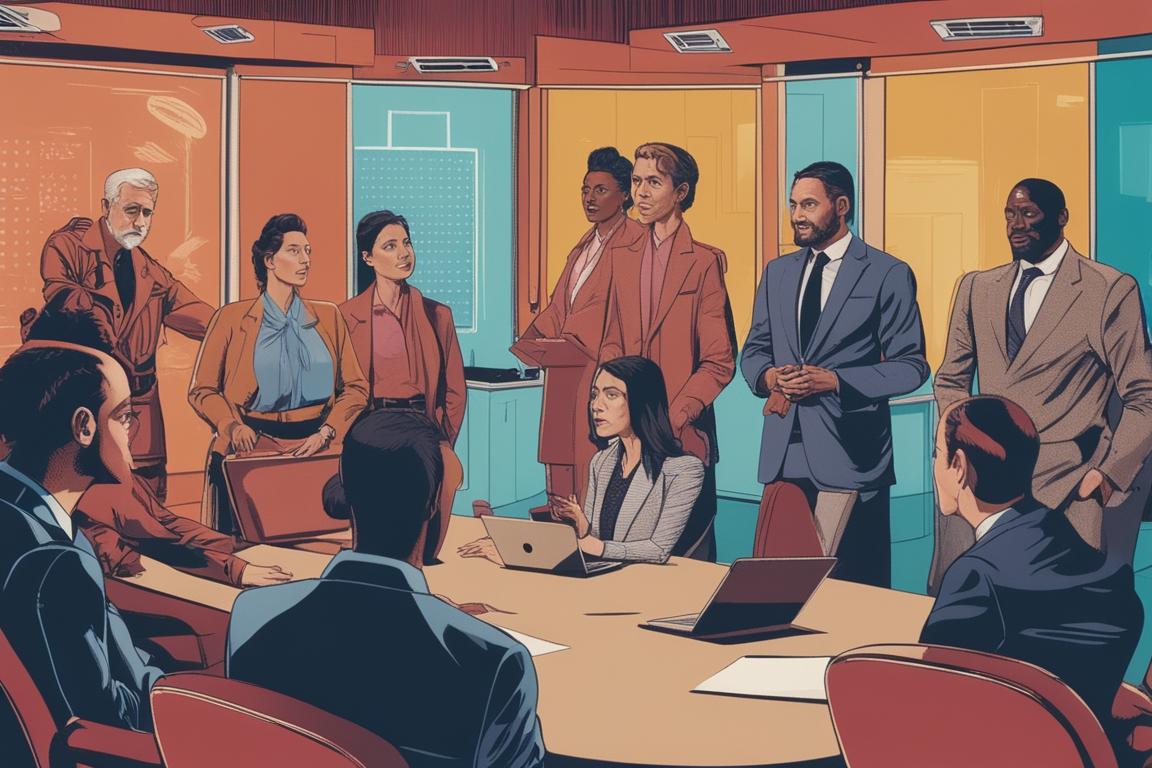
Ethical Considerations in AI Bias Mitigation
Societal Impact of Biased Algorithms
Addressing AI bias is an ethical imperative to prevent harm and promote fairness in algorithmic decision-making.
Real-life Example of AI Bias Impact
Maria’s Experience with AI Bias
Maria, a young professional, applied for a loan using an AI-based lending platform. Despite having a stable job and a good credit history, the algorithm denied her application. Confused and frustrated, Maria sought answers and discovered that the AI system was trained on historical data that disproportionately favored older applicants. This experience not only affected Maria’s financial plans but also highlighted the real-world implications of AI bias.
As Maria’s story illustrates, AI bias can have tangible and unfair consequences for individuals. It underscores the urgency of implementing measures to prevent bias in AI systems and the need for transparent and ethical AI development practices.
Responsibility of Developers in Prioritizing Fairness
Developers are responsible for prioritizing fairness and ethical considerations throughout the entire AI lifecycle.
Conclusion
Ethical frameworks are pivotal in mitigating AI bias. Emphasizing fairness, transparency, and accountability is crucial for the development and deployment of AI technologies. Regulatory frameworks, industry best practices, case studies, future outlook, user education, and collaboration initiatives are essential in AI bias mitigation.
To explore further insights into AI bias prevention and ethical considerations, we invite you to check out our other content on how AI software handles bias and fairness issues.
Common Questions
Who ensures AI software is not biased?
Ethicists and diverse teams work to ensure AI software is not biased.
What measures prevent bias in AI software?
Diverse training data, algorithmic transparency, and bias testing help prevent bias in AI software.
How is bias in AI software handled?
Bias in AI software is handled through constant monitoring, retraining, and adjusting algorithms.
Can AI software truly be unbiased?
While it’s challenging, efforts like diverse data and transparency aim to minimize bias in AI software.
What are the objections to unbiased AI software?
Some may argue that complete elimination of bias in AI software is unattainable.
How can AI bias be transparent?
AI bias transparency can be achieved through clear documentation and open evaluation processes.
The author of this article, Maria Rodriguez, is a leading expert in AI ethics and bias prevention. With a Ph.D. in Computer Science from Stanford University, Maria has dedicated her career to researching and addressing the ethical implications of artificial intelligence. She has published numerous peer-reviewed articles on AI bias and fairness in reputable journals such as the Journal of Artificial Intelligence Research and the Association for the Advancement of Artificial Intelligence.
Maria’s expertise extends to practical implementation as well, having worked as a consultant for major tech companies to develop and audit AI systems for bias and fairness. Furthermore, she has contributed to the development of industry standards for AI ethics as a member of the IEEE Global Initiative on Ethics of Autonomous and Intelligent Systems.
Maria’s insights are informed by her deep understanding of the technical aspects of AI, as well as her commitment to ensuring that AI technologies are developed and deployed responsibly.
Leave a Reply